It is difficult to imagine modern industries without artificial intelligence. While AI is not very widespread as of now, you will be surprised to learn that most businesses incorporate this technology in one way or another.
AI is something that already influences modern companiesб and using this technology will be essential moving forward. Building AI infrastructure for your company from scratch is rather challenging. Read along to find out about the challenges of implementing an AI infrastructure and using it as a service.
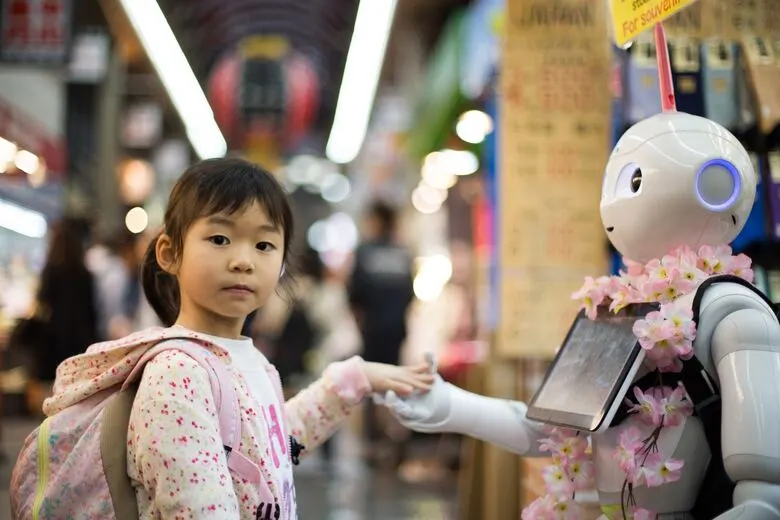
Table of Contents
Difficulties with Implementing AI
Many businesses start thinking about implementing AI in their operations to improve various processes. While AI is a revolutionary technology that allows companies to grow and achieve new heights, it is still difficult to implement it. Here are some of the most common challenges:
· Choosing the data set — AI works on the basis of quality data gathered from a company. It might be challenging to choose a fitting data set for your infrastructure.
· Data storage — AI works through large quantities of data to learn and improve. Storing all this information requires a lot of space that companies might not have right away.
· Privacy — security issues can be present when AI has access to the company data. It is crucial to implement security solutions to avoid these issues.
· Infrastructure — when working with traditional technical solutions within a company, it takes a lot of preparations to switch to AI. There should be a powerful infrastructure in place to ensure that everything operates smoothly.
GCore is a company that offers leading AI infrastructure solutions as a service. There is no need to build a whole infrastructure from scratch as you can implement Graphcore IPUs and utilize cloud solutions as well. Benefit from great flexibility and improved performance when incorporating AI cloud solutions in your company.
FAQs
What are the three problems of artificial intelligence?
Data quality and bias: AI algorithms require vast amounts of data to learn and improve, but the quality of the data used can have a significant impact on the accuracy and fairness of the results. Biases in the data, such as the underrepresentation of certain groups or over-representation of others, can lead to discriminatory outcomes. Additionally, malicious actors can manipulate data to intentionally introduce biases and subvert AI systems.
Explainability and transparency: AI algorithms can produce complex and opaque results, making it difficult to understand how they arrived at a particular decision. This lack of transparency can be problematic in many scenarios, such as healthcare or legal contexts, where the reasoning behind a decision must be explained. Furthermore, it is challenging to determine the root cause of errors or biases in an AI system without clear explanations.
Safety and security: As AI is increasingly integrated into critical systems, such as self-driving cars, healthcare, and finance, safety, and security concerns are becoming more significant. AI systems can be vulnerable to hacking, data breaches, and other cyber threats, which can lead to significant harm. Additionally, the potential for unintended consequences or unforeseen interactions between AI systems and humans could have dire consequences. Ensuring the safety and security of AI systems is a crucial challenge that needs to be addressed to maximize the benefits of AI while minimizing risks.
What are the 4 risks of artificial intelligence?
There are numerous risks associated with artificial intelligence that we face in our daily lives. Not every AI issue is as serious and concerning as killer robots or sentient AI. Consumer privacy, biased programming, human danger, and unclear legal regulation are some of today’s most serious threats.
Leave a Reply